- Shining on the top spot in Hugging Face’s leaderboard of instruction-tuned LLMs
- It stands out for two primary reasons: it offers a commercial license and delivers exceptional performance.
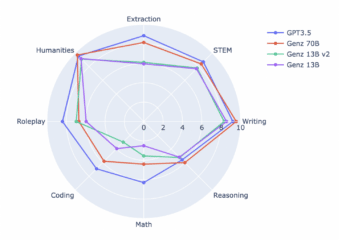
Accubits Technologies has introduced GenZ 70B, their fifth large language model (LLM), which has been open-sourced. It is an instruction-fine-tuned model that offers a commercial licensing option and is ranked sixth among all open LLMs in various categories. This marks a significant milestone, representing the first such achievement originating from India.
An advanced LLM has undergone a fine-tuning process using Meta’s open-source Llama-2 70B parameter model. This fine-tuning was primarily conducted to enhance its reasoning, role-playing, and writing capabilities. The decision to utilise Llama-2 was driven by its status as a pre-trained model architecture, surpassing other commercially available open-source LLMs. This model incorporates RoPe positional embedding, allowing for context interpolations, which means the model’s contextual understanding can be extended as needed. It also incorporates attention mechanisms like ghost, enhancing memory, computational efficiency, and alignment and has already been pre-trained on an impressive 2 trillion tokens.
Outperforming competing LLMs
In initial evaluations, the model scored 70.32 on the MMLU benchmark (Measuring Massive Multitask Language Understanding), surpassing LLama-2 70B’s score of 69.83. It achieved a score of 7.34 on the MT (multi-turn) benchmark. While many fine-tuned models exist, most do not provide commercial licenses. These models have undergone refinement through the supervised fine-tuning (SFT) technique, selected after multiple experiments identified SFT as the optimal choice. Parameter Efficient Fine-tuning (PEFT) methods are employed for fine-tuning LLMs. They do not perform well for long-term, multi-stage fine-tuning as accuracy tends to degrade with each stage, eventually leading to catastrophic forgetting and model drift. They have observed that PEFT methods significantly impact the model’s generalisation capability more than supervised fine-tuning.
Their primary focus was on instruct-tuning the model to enhance its reasoning, role-playing, and writing proficiencies. This approach enables a wide range of use cases and business applications, including but not limited to business analysis, risk assessment, project scoping, and conversational tools. They believe organizations can leverage GenZ 70B to tackle specialized challenges and foster innovative solutions. Smaller quantisation versions of GenZ models make them accessible, facilitating their utilization even on personal computers. Within the open-source community, there are three models with varying parameter counts (7B, 13B, and 70B) and two quantization options (32-bit and 4-bit) at users’ disposal.
Constraints persist
The model’s creators have advised caution when considering its deployment for production purposes. Like other large language models (LLMs), its 70B is based on extensive web data, which means it may exhibit online biases and stereotypes. They recommend users consider fine-tuning it to suit their specific tasks of interest. The company’s blog also emphasizes the importance of using precautions and safeguards in production environments.
With this model, the company is on a mission to develop open-source foundational models with the knowledge and reasoning capabilities of GPT-4, which strongly emphasises privacy and can even be hosted on a personal laptop. Their vision is driven by the belief that the power of LLMs should be accessible to all, not limited to a privileged few, to facilitate the collective advancement of society. After all, technological progress realizes its full potential when a diverse range of users can harness it.