Artificial intelligence for IT operations or AIOps is a boon for the modern day enterprise. Read on to find out its benefits, and get to know the popular AIOps platforms.
The adoption of new business models by an enterprise due to digital transformation has increased the complexity of IT operations (ITOps). As a result, existing IT tools can no longer meet enterprise demands. The biggest challenges ITOps face today are:
- Poor user experience on applications across geographies
- Lack of effective event management and no proactive monitoring
- More manual intervention and efforts, and minimum automation
- Inability of component-level drill down across technology platforms for a quick problem and root cause analysis
- Lack of single-pane view of IT and business process metrics
Organisations have now started leveraging artificial intelligence (AI) for IT operations (AIOps) with APM (application performance monitoring) and other data sources to gain insights that improve business outcomes.
AIOps is the use of artificial intelligence (AI) for IT operations to enhance, support and automate the latter. It covers the strategic use of AI, analytics, and machine learning (ML) technologies across IT operations to simplify and streamline processes and optimise the use of IT resources.
It can be considered as a platform consisting of AI and ML engines, Big Data capabilities, and servers covering storage, compliance, infrastructure, provisioning, and backup. The AIOps platform helps in:
- Improving and automating event monitoring
- Ingesting both historical data and real-time streaming data from across the IT environment
- Filtering out the noise so only the most relevant data is analysed
- Better service management
- Modernising IT operations
- Implementing security operations (SecOps), network operations (NetOps) and development operations (DevOps) by using AI to automate IT
Industry adoption of AIOps
According to ESC Research, nearly 30 per cent of the organisations surveyed plan to make significant investments in AIOps over the next 12 to 18 months, and more than 90 per cent expect to spend as much or more on AI and machine learning in 2023.
Gartner predicts that the number of business leaders relying on AIOps platforms for automated insights will increase 10 times by 2024.
As per an IDC report, by 2024, 30 per cent of enterprises will extend attention networks across IT teams, including AIOps.
According to Research and Markets, the global market for AIOps platforms is projected to reach US$ 22.9 billion by 2030, growing at a CAGR of 30.4 per cent between 2022 and 2030.
The key objectives of AIOps are (Figure 1):
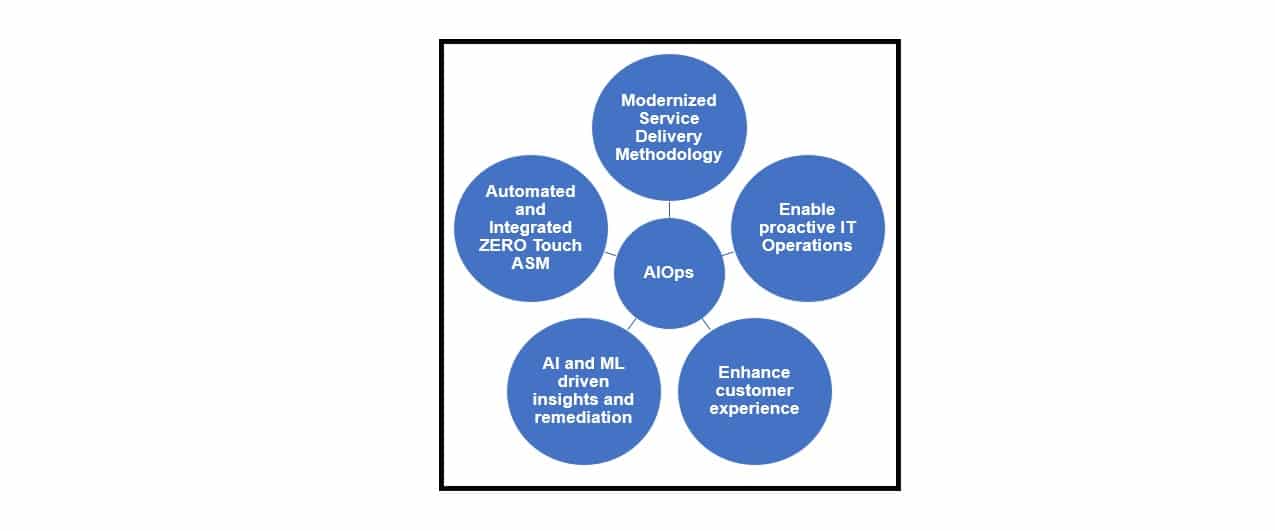
- Monitoring
- Event correlation
- Auto ticketing
- Anomaly detection
- Business IQ
- Business transactions monitoring
- Auto remediation
- RCA/Diagnostics
The AIOps framework
AIOps services focus on optimisation, simplification, automation, and elimination to improve the resilience of IT systems, leading to an enhanced customer experience. The AIOps framework enables proactive end-to-end business — IT monitoring and analytics, next-gen event management, and robotic process automation (Figure 2). The aim is to simplify IT operations.
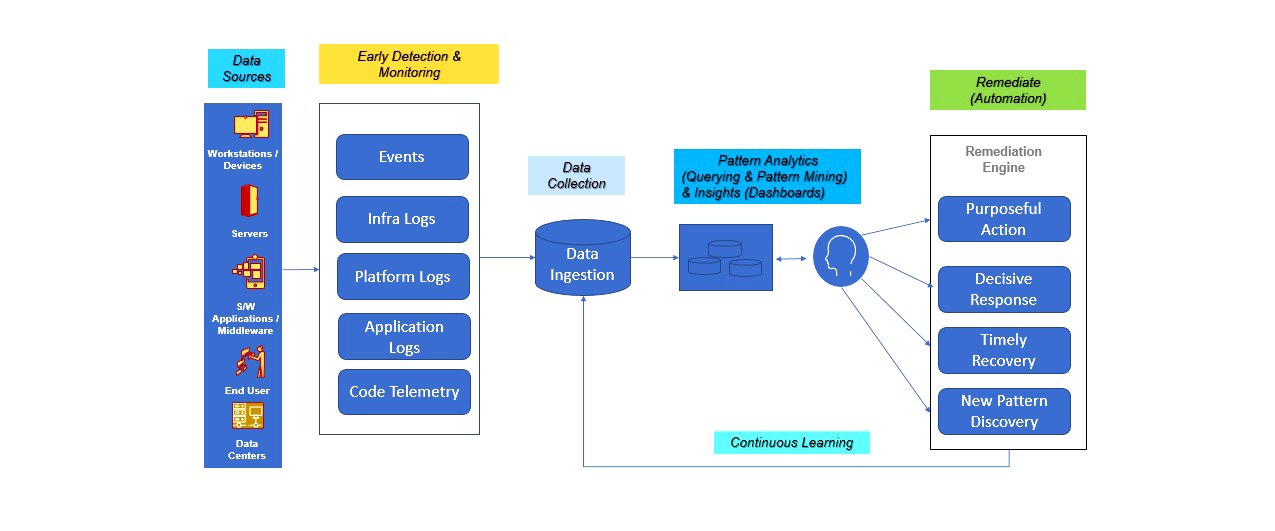
Let’s take a look at the components of the framework of an AIOps platform.
Early detection: It is important to continuously monitor all business-critical applications for availability and performance. A significant amount of time and effort is spent on activities such as application monitoring, batch monitoring, etc. Early detection helps in:
- Early warning of potential performance bottlenecks
- Increased application accessibility for a better user experience
Data collection: A key aspect of AIOps is comprehensive analytics that leads to actionable insights. This involves ingestion of data from multiple sources that are vendor-agnostic, storage of the acquired data, real-time analysis at the point of ingestion and historical analysis of stored data, leveraging machine learning and, finally, preventive and remedial actions based on the analysis.
Pattern analytics: This offers real-time analysis and visualisation of automatically collected and correlated data to get insights into IT operations, customer experience and business outcomes. It helps to:
- Analyse rich and extensible data sets to connect the dots between IT operations, user experience and business impact
- Easily collect and correlate user, performance and business data in real-time with no code changes
- Utilise SQL on rich and extensible data sets to run ad hoc analysis in real-time and dig deeper into specific performance issues
These insights provide a holistic view of business process and application metrics, which gets generated from different monitoring and automation systems.
Remediation: This helps to reduce the effort put in tasks related to tickets, which can be automatically addressed via orchestrated platforms and self-service solutions. Remediation helps in:
- Reduction of execution time for tasks/processes
- Increased operational efficiency with reduced mean time to resolution
Open source AIOps platforms
Most open source AIOps projects use Python as a programming language for machine learning. Based on the enterprise requirement, various AIOps and open source tools can be combined and used on AIOps platforms. We take a brief look at the top open source AIOps platforms/tools.
SeldonIO: This open source platform deploys enterprise machine learning models on Kubernetes at a massive scale. Seldon handles scaling to thousands of production machine learning models and provides advanced machine learning capabilities out-of-the-box, including advanced metrics, request logging, explainers, outlier detectors, A/B tests, canaries, and more.
Logpai/Loglizer: This is a machine learning-based log analysis toolkit for automated anomaly detection. Loglizer provides a toolkit that implements a number of ML-based log analysis techniques that have multiple supervised and unsupervised models.
Whylabs/Whylogs: Whylogs is an open source statistical logging library that allows data science and ML teams to effortlessly profile ML/AI pipelines and applications, producing log files that can be used for monitoring, alerts, analytics, and error analysis. It’s available in Python and Java.
Jixinpu/Aiopstools: This fundamental package for AIOps with Python offers the following:
- Anomaly detection
- Alarm convergence
- Time series forecasting method
- Association analysis for alarms
Log-anomaly-detector: This platform is developed using ML, and is used for log anomaly detection by connecting to streaming sources to predict abnormal log lines. It uses unsupervised machine learning models to achieve this result.
Prometheus: This open source monitoring solution simplifies pulling numerical metrics from a metrics endpoint.
Grafana: This open source metric analytics and visualisation suite is popular among Prometheus users to visualise the metrics.
Elastic Stack: This is a suite of open source products from Elastic designed to help users search, analyse, and visualise data from any type of source, in any format, in real-time. It provides monitoring and logging solutions.
Benefits of AIOps adoption
- Improved application availability and customer satisfaction.
- Minimised application downtime on even the busiest and highest transaction days
- Expensive service disruptions are avoided, and firefighting eliminated
- Continual management of vulnerability risks. AIOps tools help to identify, analyse, prioritise and remediate vulnerability risks
- Intelligent alerts to prevent potential issues
- Increased business responsiveness
- Helps make data-driven decisions
- Helps to meet growing business demands
- Increased efficiency and optimised running costs with the help of automation and AI
- Reduced human intervention and efforts; focus on innovation
The goal of AIOps is automation, which helps in simplifying administrative tasks, thus saving time. An AIOps platform helps to scale up IT operations to support ever-growing business demands, ensuring an enhanced customer experience. It reduces the complexity of IT operations by streamlining systems, configuration management, simplifying operations and improving reliability. In short, it helps to enhance the performance of enterprise operations.
Dislaimer: The views expressed in this article are that of the author and HCL
does not subscribe to the substance, veracity or truthfulness of the said opinion.