AI-augmented decision making is making a transformative impact across various fields, benefiting a range of industries.
Artificial intelligence (AI) is evolving rapidly, with various technologies converging to create more sophisticated and versatile systems. The adoption of AI-augmented decision making has resulted in numerous benefits across various fields, including improved accuracy, efficiency, and cost savings. By leveraging AI algorithms to analyse vast amounts of data and generate actionable insights, organisations can make more informed decisions, optimise processes, and enhance productivity. Moreover, AI-driven predictive analytics enables proactive interventions and personalised solutions.
AI-augmented decision making has emerged as a transformative force across diverse fields, revolutionising how organisations analyse data, make decisions, and achieve their objectives. As AI continues to advance, its impact on decision making is expected to grow, unlocking new opportunities and transforming industries in profound ways. Embracing AI-augmented decision making will be essential for organisations seeking to thrive in an increasingly competitive and data-driven world.
The concept of human-AI collaboration
Human-AI collaboration is a mutually beneficial association between intelligent machines and humans, in which both entities utilise their distinct capabilities to accomplish shared objectives. This approach, in contrast to conventional notions of automation supplanting human labour, emphasises the complementary nature of AI and human capabilities.
Artificial intelligence (AI) systems demonstrate exceptional proficiency in the processing of enormous volumes of data, identification of patterns, and execution of repetitive tasks with accuracy and velocity. However, it is difficult for artificial intelligence to emulate the creative abilities, intuition, empathy, and complex problem-solving skills possessed by humans. Human-AI collaboration maximises the combined capabilities of both entities, resulting in improved decision-making, problem-solving, and innovation in a multitude of fields.
AI-augmented decision-making in various fields
Healthcare: In the healthcare sector, AI-augmented decision making has revolutionised diagnosis, treatment planning, and patient care. AI algorithms analyse medical images, such as X-rays and MRIs, with unparalleled accuracy, aiding radiologists in detecting abnormalities and making diagnostic decisions.
Finance (financial services, insurance, capital markets and banking): In finance, AI plays a crucial role in risk management, fraud detection, and investment strategies. AI algorithms analyse market trends, economic indicators, and historical data to predict stock price movements and optimise investment portfolios. Furthermore, AI-powered chatbots and virtual assistants provide personalised financial advice to customers, enhancing user experience and engagement.
Manufacturing: AI-augmented decision making is transforming manufacturing processes, enabling predictive maintenance, quality control, and supply chain optimisation. Predictive maintenance systems powered by AI analyse equipment sensor data to predict potential failures and schedule maintenance proactively, minimising downtime and reducing costs.
Marketing and retail: In the marketing and retail sectors, AI-driven analytics empower businesses to understand consumer behaviour, personalise marketing campaigns, and optimise pricing strategies. AI algorithms analyse customer data from various sources, including social media, online interactions, and purchase history, to identify trends and preferences. This enables businesses to tailor their marketing messages and offerings to individual customers, enhancing customer satisfaction and loyalty. Moreover, AI-powered recommendation systems provide personalised product recommendations, increasing sales and revenue.
Transportation: AI-augmented decision making is reshaping the transportation industry, enabling autonomous vehicles, route optimisation, and predictive maintenance. Autonomous vehicles leverage AI algorithms to interpret sensor data, navigate complex environments, and make real-time driving decisions. Route optimisation systems powered by AI analyse traffic patterns, weather conditions, and historical data to recommend the most efficient routes for vehicles, reducing fuel consumption and emissions.
Enhancing creativity and innovation through AI
The intersection of artificial intelligence and human creativity can augment and inspire innovation across various domains. Here’s a broad overview along with examples.
AI-powered idea generation: AI algorithms can analyse vast amounts of data to identify patterns and insights that humans might overlook. For example, in marketing, AI can analyse consumer behaviour data to suggest novel product ideas or marketing strategies.
Platforms like OpenAI’s GPT-3 can generate creative content, such as writing poetry, composing music, or designing artworks, based on prompts provided by users.
Assisting creative processes: AI tools can assist in various stages of the creative process. For instance, Adobe’s Photoshop and Illustrator use AI algorithms to suggest design elements or enhancements based on user inputs.
In film production, AI can aid in tasks like scriptwriting, storyboarding, and even generating visual effects.
Facilitating collaborative innovation: AI-powered collaboration platforms can bring together diverse teams to brainstorm and innovate collectively. These platforms leverage AI to facilitate idea sharing, project management, and feedback gathering, enabling teams to work more efficiently.
For example, Slack’s integration with various AI-powered bots and plugins streamlines communication and project management, fostering collaboration among team members.
Predictive analytics for innovation: AI-driven predictive analytics can anticipate future trends and market demands, providing valuable insights for innovative product development and business strategies.
Companies like Netflix and Spotify use AI to analyse user preferences and behaviour patterns to recommend personalised content, thereby driving innovation in content delivery and curation.
Automating repetitive tasks: By automating mundane tasks, AI frees up human creativity and cognitive resources for more innovative endeavours. For example, AI-powered chatbots can handle customer enquiries, allowing human customer service agents to focus on solving complex issues and devising creative solutions.
Enhancing decision making: AI algorithms can analyse large datasets and provide insights to support decision-making processes. This capability is particularly valuable in fields like finance, healthcare, and manufacturing.
For instance, in drug discovery, AI models can analyse molecular structures and predict the efficacy of potential drugs, accelerating the innovation process and reducing time-to-market.
Psychological and societal aspects of human-AI interaction
Human-AI collaboration is predicated on the physiological interaction that takes place between machines and humans. Augmented reality (AR) systems, brain-computer interfaces (BCIs), voice commands, and gesture recognition all facilitate seamless communication and interaction. BCIs facilitate direct communication between artificial intelligence (AI) systems and the human brain, thereby enabling users to manipulate devices or express concepts without requiring physical movement. Likewise, augmented reality (AR) technologies superimpose digital data onto the tangible environment, leading to data visualisation that augments human capabilities. In addition to enhancing productivity, these physiological interfaces facilitate accessibility for people with disabilities, thereby fostering more inclusive environments.
However, the collaboration between humans and AI gives rise to significant social concerns. It is imperative to confront ethical dilemmas pertaining to privacy, data security, and AI bias to foster collaboration that is equitable and transparent. Proactive strategies to alleviate prospective inequalities are imperative due to the effect that AI will have on employment and socioeconomic structures.
The establishment of trust between human beings and AI systems is also imperative to facilitate productive collaboration. Establishing trust and user acceptance relies heavily on several key elements: transparency in AI decision-making processes, explainable AI algorithms, and explicit communication regarding the capabilities and limitations of AI. By fostering inclusivity and diversity within AI development teams, biases can be reduced and AI systems can be designed to meet the requirements of a wide range of users.
Envisioning the future of work with AI augmentation
The relationship between AI and human labour is evolving continuously, and promises to enhance and transform various aspects of work.
AI-powered automation: AI-driven automation is reshaping traditional job roles by streamlining repetitive tasks and processes. For example, in manufacturing, AI-enabled robots can handle assembly line operations with speed and precision, reducing the need for manual labour. In administrative tasks, AI-powered chatbots and virtual assistants can handle customer enquiries, schedule meetings, and perform data entry, freeing up human workers to focus on more complex and creative aspects of their jobs.
Augmented intelligence: Rather than replacing human workers, AI augmentation aims to enhance human capabilities by providing real-time insights and decision support. For instance, in healthcare, AI algorithms can analyse medical images to assist radiologists in detecting abnormalities more accurately and efficiently. In financial services, AI-powered predictive analytics tools can help investment professionals identify market trends and make informed decisions, augmenting their expertise with data-driven insights.
Personalised learning and development: AI technologies can personalise learning experiences for employees, identifying their skill gaps and providing tailored training programs. For example, AI-powered learning platforms can recommend relevant courses and resources based on an individual’s job role, career goals, and learning preferences. Through adaptive learning algorithms, employees can acquire new skills and knowledge at their own pace, enabling continuous professional development in a rapidly changing work environment.
Collaborative intelligence: AI collaboration tools facilitate teamwork and knowledge sharing among distributed teams. For instance, virtual collaboration platforms equipped with AI-powered chatbots, project management tools, and collaborative document editing capabilities enable seamless communication and coordination. By leveraging AI for real-time language translation and transcription, global teams can overcome language barriers and collaborate effectively across borders, fostering innovation and diversity in ideas.
Ethical considerations and human-centric design: As AI becomes more integrated into the workplace, it’s essential to prioritise ethical considerations and ensure that AI technologies align with human values and goals. This includes addressing concerns related to privacy, bias, and job displacement.
Human-centric design principles should guide the development and deployment of AI systems, prioritising transparency, accountability, and user empowerment. Workers should have agency over AI tools, with mechanisms in place to mitigate potential risks and ensure fair treatment.
Reskilling and adaptability: The widespread adoption of AI augmentation necessitates a focus on reskilling and upskilling initiatives to prepare the workforce for the jobs of the future. Organisations need to invest in training programs that equip employees with the digital skills and technological literacy required to thrive in an AI-augmented workplace.
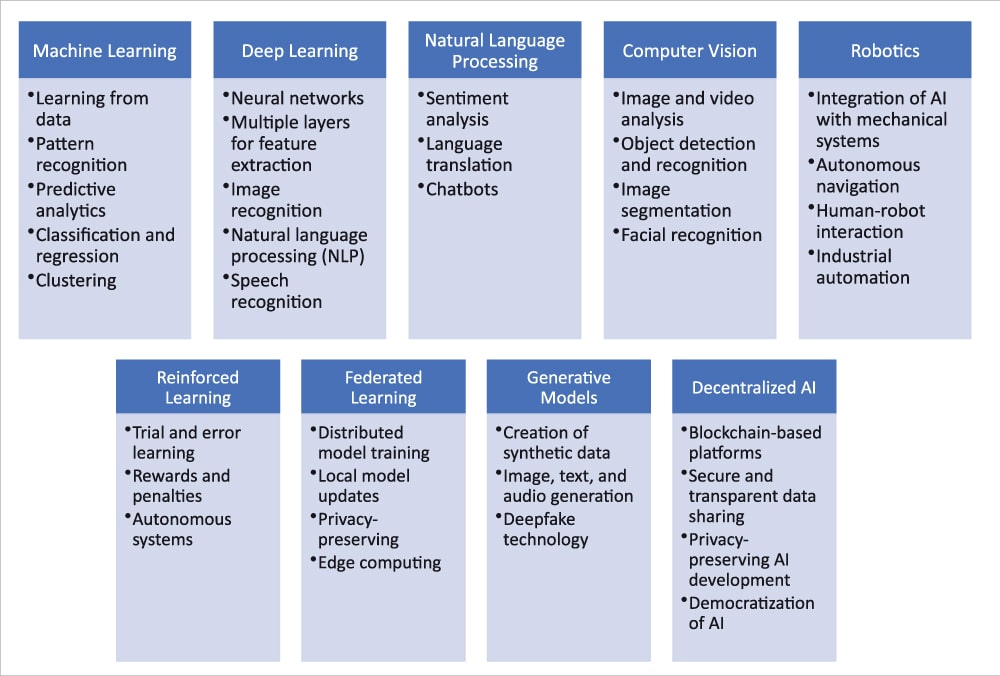
Convergence of AI technologies
Human-AI collaboration and augmentation are perpetually progressing due to the convergence of numerous AI technologies. The convergence of machine learning algorithms, natural language processing (NLP), computer vision, robotics, and decentralised AI systems is facilitating the development of increasingly intelligent and flexible AI solutions. As machine learning models improve their ability to comprehend and produce natural language, they facilitate human-machine interaction that is more intuitive in nature. AI systems are enabled by computer vision technologies to interpret and react to visual stimuli, which facilitates the execution of activities including autonomous navigation, image classification, and object recognition.
Machine learning and deep learning
Machine learning (ML) and deep learning (DL) are foundational components of AI that enable systems to learn from data and make predictions or decisions. ML algorithms, such as decision trees and support vector machines, analyse data to identify patterns and relationships. Deep learning, a subset of ML, utilises neural networks with multiple layers to extract intricate features from complex data, enabling tasks such as image recognition, natural language processing (NLP), and speech recognition. The convergence of ML and DL techniques has led to breakthroughs in AI applications, from autonomous vehicles to medical diagnostics.
Natural language processing (NLP) and generative models
NLP allows AI systems to understand, interpret, and generate human language, enabling applications such as chatbots, language translation, and sentiment analysis. Generative models, such as generative adversarial networks (GANs) and transformers, can create realistic text, images, and audio, revolutionising content creation and synthesis. The convergence of NLP and generative models has paved the way for advancements in conversational AI, content generation, and creative applications, such as deepfake technology and virtual assistants.
Computer vision and robotics
Computer vision enables AI systems to analyse and interpret visual data, including images and videos, facilitating tasks such as object detection, image recognition, and autonomous navigation. Robotics integrates AI with mechanical systems to create intelligent machines capable of sensing, perceiving, and interacting with the physical world. The convergence of computer vision and robotics has fuelled advancements in autonomous vehicles, industrial automation, and healthcare robotics.
Reinforcement learning and autonomous systems
Reinforcement learning (RL) is a branch of ML that enables AI agents to learn optimal behaviour through trial and error, receiving rewards or penalties based on their actions. RL algorithms power autonomous systems, such as self-driving cars and drones, enabling them to navigate dynamic environments and make real-time decisions. The convergence of RL with other AI technologies, such as computer vision and NLP, enhances the capabilities of autonomous systems, enabling them to perceive and interact with their surroundings more effectively.
Federated learning and decentralised AI
Federated learning is a distributed ML approach, where model training occurs locally on decentralised devices, such as smartphones or edge devices, and aggregated results are used to improve a global model. This enables collaborative learning without centralised data aggregation, preserving user privacy and data security. The convergence of federated learning with decentralised AI systems, such as blockchain-based platforms, has the potential to democratise AI development and deployment, enabling secure, privacy-preserving AI applications across various domains.
As AI systems become more sophisticated and versatile, they will play increasingly prominent roles in healthcare, finance, transportation, manufacturing, and beyond. However, this convergence also raises important ethical, social, and regulatory considerations, including data privacy, algorithmic bias, and accountability. Moving forward, it will be crucial to ensure that AI technologies are developed and deployed responsibly, with a focus on fairness, transparency, and inclusivity.