Using Speaker Diarization AI Technology, Google is able to partition audio stream including multiple voice inputs from different people and claim 92% accuracy.
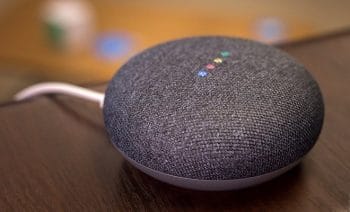
In a recent blog post, Google announced that they have open-sourced their speaker diarization technology, which is able to differentiate people’s voices at a high accuracy rate.
Speaker diarization is a process of partitioning an audio recording having voices of multiple people into distinct segment associated with each individual. It is an important part of speech recognition systems.
This AI technology solves the problem of “who spoke when” and it has applications in many important scenarios, such as understanding medical conversation, video captioning and more.
The challenges
Google noted that training voice dictation systems with supervised learning methods is challenging.
In the blog, Chong Wang, Research Scientist at Google AI explains: Unlike standard supervised classification tasks, a robust diarization model requires the ability to associate new individuals with distinct speech segments that weren’t involved in training. Importantly, this limits the quality of both online and offline diarization systems. Online systems usually suffer more, since they require diarization results in real time.
Google has developed a research paper called Fully Supervised Speaker Diarization where they have described a new model that uses supervised speaker labels in a more effective manner.
On the NIST SRE 2000 CALLHOME benchmark, Google’s techniques achieved a diarization error rate (DER) as low as 7.6 percent, compared to 8.8 percent DER from its previous clustering-based method, and 9.9 percent from deep neural network embedding methods.
Now, Google has open sourced the core algorithms and made it available on Github to accelerate more research along this direction.
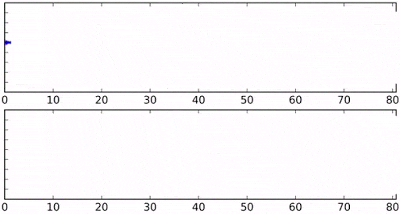
Difference between Google’s model and common clustering algorithms
In Google’s method, all speakers’ embeddings are modeled by a parameter-sharing recurrent neural network (RNN), and different speakers are distinguished using different RNN states, interleaved in the time domain. Each speaker starts with its own RNN instance (with a common initial state shared among all speakers) and keeps updating the RNN state given the new embeddings from this speaker.
Representing speakers as RNN states enables the system to learn the high-level knowledge shared across different speakers and utterances using RNN parameters. This promises the usefulness of more labeled data. In contrast, common clustering algorithms almost always work with each single utterance independently, making it difficult to benefit from a large amount of labeled data.
Future plan
Google plans to refine the model so that it can integrate contextual information to perform offline decoding, with further reduced DER. The tech giant is also planning to add some acoustic features to train entire speaker diarization system in an end-to-end way.