Why do patients have to wait for hours to get tested when artificial intelligence (AI) can do it in seconds? Read on to learn how AI and machine learning skills can result in solutions to emerging problems, particularly during a pandemic.
Priya’s mother had a fever and a sore throat. Worried that these could be symptoms for COVID-19, she decided to get her mum tested at the nearest public health centre (PHC). When they reached the PHC, the duo froze for a moment, in shock. There were at least a 100 others, waiting in a queue to be tested as well. It took over six long hours for their turn and to complete the tests. “Wouldn’t being exposed to so many people here make us vulnerable to new infections?” Priya asked her mum. “If one wants to get tested, I suppose we have to put up with this,” the duo thought. Little did they know that there are AI-powered chatbots that can perform the same preliminary tests, in the comfort and safety of one’s homes. Patients do not have to go in person to crowded public places to get a confirmed diagnosis.
Chatbots for COVID-19 screening
Chatbots are no longer preprogrammed rule-based algorithms that render stock answers. Machine learning (ML), natural language processing (NLP) and artificial intelligence (AI) capabilities are now transforming chatbots, embedding them with a certain degree of ‘intelligence’ to understand the user’s language and search intentions. Deep learning models of Python, such as Universal Sentence Encoders (USE), perform effective text vectorisation so that texts can be understood both for context and semanticity.
For example, let’s look at a scenario in which one user asks, “What are the symptoms of COVID-19?” another user types “Corona symptoms”, and a third user, “I have a running nose. Is it Corona?” Now, all three questions, though differently worded, have the same meaning. USEs and NLPs understand these language variations and semanticity, and can render direct answers relevant to the user’s search intent. Also, chatbots can perform automated preliminary health screenings by deploying forms on websites or mobile applications. Direct answers and test results can be rendered in a matter of seconds.
Neural networks for monitoring progression
Medical imaging like X-rays, CT scans, mammograms, etc, have become fundamental to healthcare monitoring. Doctors assess disease progression using radiology expert analysis and scan reports. Neural networks and the deep learning algorithms of AI can go a long way in accelerating the speed of the analysis and auto-generating the scan reports. Image-to-image neural networks use the new images (the input layer) with their internally trained disease data sets (hidden layer) to generate output images (output layer). These neural networks work on feed forward and back propagation mechanisms to thoroughly investigate the raster image data sets.
There are two benefits of adapting to neural networks based disease monitoring. First, conventionally, scanning machines use high radiation exposure in patients to generate high quality or clear scanned images. High-quality scanned images came at the cost of a patient’s health. High radiation poses the risk of cancer and it has been considered an inevitable side-effect for those who take scans. However, with image-to-image neural networks, it is now possible to let the patient get scanned with minimum radiation exposure. Low quality captures, distorted images, or other minuscule errors (termed as data noise) will work. The deep learning algorithms compare the input images with their internally trained mass classifier data sets, to auto reduce all data noise. Neural networks are trained to recognise noise signature distributions, improve image resolutions, enhance image contrasts and provide a more accurate analysis of the disease’s progression.
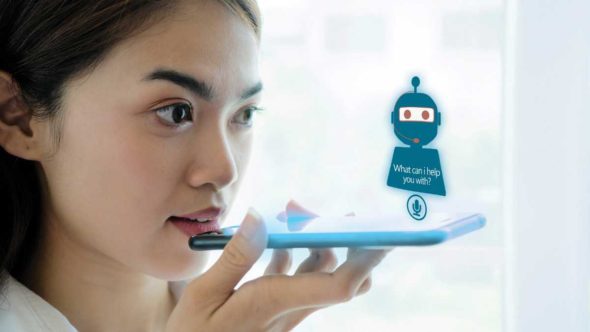
The second benefit is the quality of the prognosis and the accuracy of the results. Radiology experts no longer need to perform a detailed subjective analysis on every scan or testing sample that comes their way — a task that is time-intensive, repetitive and costly. A neural network can do the same job with greater accuracy (up to 95 per cent) in just a few seconds. With the ongoing COVID-19 pandemic, patients with respiratory illnesses like pneumonia wait to get themselves scanned and see the results. However, a few hospitals implement applications like qXR to calculate the risk of COVID-19 infections using neural networks. Smart AI solutions help them get a quicker diagnosis of disease progression. The swift, timely results also cascade into other healthcare processes, like providing prompt medical interventions and immediate treatment, thereby increasing the likelihood of recovery. For active disease control, especially during pandemics, neural networks can deliver test results in a much more robust and faster way.
Automation for disease tracking
Public health care systems have been around since the last decade. All hospitals in India have automated patient records, but for administration and billing purposes only.
But, what if we could extend this automation to the individual level to archive medical histories and track recoveries? What if disease tracking went beyond the walls of the physical hospitals?
Today we have tons of fitness applications that track a patient’s pulse, BMR, diet, exercise, sleep, water intake, and other vital biological statistics. These applications integrate smart watch sensor readings with mobile widgets to populate user details. Automation can work along similar lines, integrating patient statistics with pre-populated medical data repositories. It can facilitate proactive patient follow-ups, monitor disease progression and holistically enhance the quality of medical services. When integrated with bio-medical sensors of smart devices, it can provide useful physiological insights to medical practitioners.
Centralised Web based disease surveillance is the need of the hour for active disease tracking of individual patients. Imagine if all doctors had access to the medical history of their patients at a click of a button, without having to manually review each of the paper files or verbal declarations. Would it not accelerate the time taken for treatment and the overall quality of medical services? Centralised disease surveillance systems are also some of the fundamental precursors to implement successful telemedicine services.
The technology that we need in order to create advanced and holistic medical automation systems already exists today. We just need someone to put together all the different aspects to create the right product.
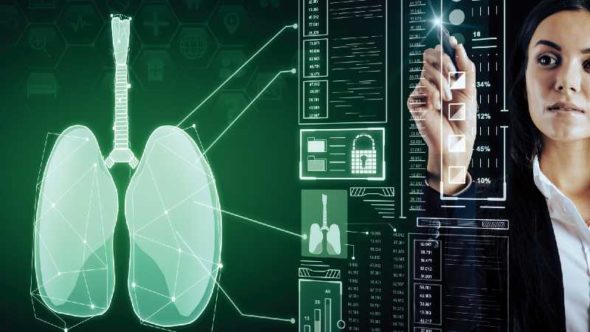
Machine learning for disease surveillance
The success of the PMO’s Aarogya Sethu mobile application can be primarily attributed to the government’s interest in solving human problems using technology. During this COVID-19 pandemic, contact tracing and disease tracking was done using the Blue Light Energy (BLE) functionality of mobile devices. Comprehensive information on infected citizens, and all those who came in proximity with them, was sent to the central server. Citizen alerts with options for preventive actions were sent to the users.
Pandemic or disease spread has a spatial and socio-demographical context. COVID-19 fatalities, for instance, are more prominent in the elderly population than other age groups. Now, what if we had software that is able to infer such trends and also pre-populate a cadastral hotspot map, with all the contact and address details of the identified elders in a specified age group? Gaining insights into such trends can help the government take appropriate disaster mitigation steps in real-time. Machine learning has the power to provide such robust insights. Not only can the deep learning algorithms predict trends, but they can also generate spatial maps and tabular reports of all vulnerable populations. These outputs can significantly help the government, epidemiologists, health care workers and other stakeholders make informed decisions that can efficiently control the disease’s spread.
Artificial intelligence and machine learning can also help enforcement officials monitor citizen compliance with public policies. Deep learning algorithms can compute the dynamic relative coordinates of moving objects or pedestrians, and even detect anomalies between them. All that is needed is a bird’s eye view input data set of the target objects. The street surveillance cameras, which serve as a bird’s eye view data set, can be integrated with Python libraries to detect pedestrian violations.
Many countries have used AI, machine learning, and the Internet of Things to monitor social distancing among citizens. The systems are able to measure the actual distances between individual pedestrians, in units of metres or kilometres, by computing the sum of relative pixel distances between them. Such AI based applications are necessary for public enforcement monitoring, and controlling disease spread.
Artificial intelligence (AI), machine learning, the Internet of Things (IoT) and Web applications have enhanced the quality of our lives. Until now, a large section of society resisted automation in the fear that AI was taking over humans. But if these advancements had already been in place, AI could have saved many human lives and better controlled the COVID-19 pandemic. We would not have to wait long in queues just to get tested, nor would the expert healthcare practitioners have had to work overtime. The AI-driven systems approach and applications could have provided the necessary technology and insights to better manage the pandemic.
AI will not take over humans. Instead, it will support them. Humans will be able to perform even better at their jobs by working alongside AI-driven systems. AI has the power to control any epidemic, not just COVID-19. Hence, in the better interest of society, let us embrace the power of AI.